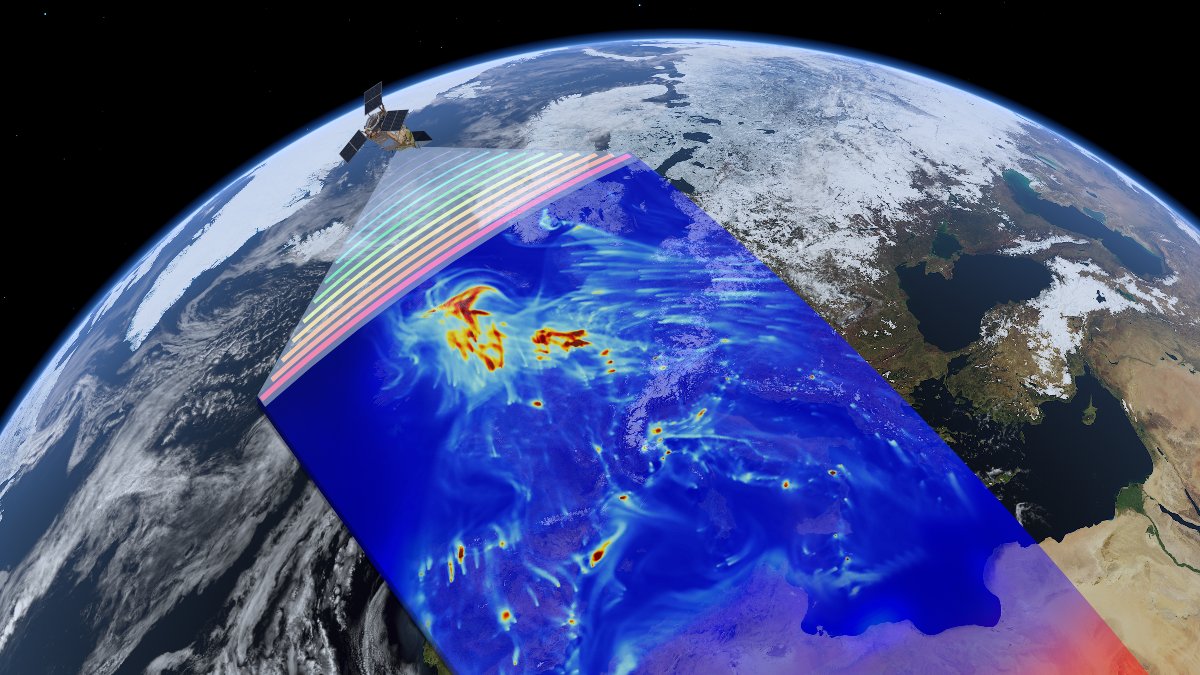
AI for Air Quality
Automated detection of atmospheric NO2 and CH4 plumes over Greater Kampala metropolitan area using high-resolution satellite observations from TROPOMI and Deep learning methods
Premium participation: Get 1-on-1 Mentoring & Guidance
Try a free mentoring sessionCreate a DL model to automatically detect NO2 and CH4 plumes/sources in Greater Kampala Metropolitan Area (GKMA)
Exposure to air pollution is one of the largest environmental health risks in Uganda. Nitrogen Dioxide (NO2) and Methane (CH4) are among the key pollutants with levels generally above the world health organisation guidelines for major cities in Uganda. The high levels of NO2 and CH4 are mainly attributed to traffic, reconditioned motor vehicles and industrial emissions. CH4 is also generated through livestock and other agricultural activities, as well as the decomposition of organic waste in landfills for solid waste. The key to efficient air quality management is quantifying pollution levels and identifying major pollution sources to allow early detection of such sources and inform air pollution mitigation efforts.
Ground monitoring of gaseous pollutants such as NO2 and CH4 is nearly non-existent in Uganda due to the high cost of setting up and maintaining a monitoring network and the unavailable skilled labour to manage the network.
NO2 and CH4 satellite observations are now possible from a number of instruments with a very high spatial resolution which allows mapping of pollutant emission plumes from individual emission sources.
Machine learning methods allow us to efficiently exploit the growing volume of satellite data available to quantify pollution levels and identify pollution sources to inform air pollution mitigation efforts.
In AI for Air Quality, we are partnering with AirQo to create a deep learning model that automatically detects NO2 and CH4 plumes in the Greater Kampala Metropolitan Area.
AirQo uses low-cost air quality monitoring sensors and machine learning algorithms to provide timely accurate, and localised air quality data to communities, governments, and organisations across various African cities, with the aim of improving public health, raising awareness of air pollution and its effects and informing policy.
Info session
In this challenge, we will
- Use remote sensing
- Do air quality/environmental monitoring
- Create Deep learning models
- Do Data analysis and Visualisation/ Data science
Technologies we will use
- Satellite data retrieval
- Deep learning
- Data visualisation
- Computer vision
Who are we looking for?
We are looking for data science & AI engineers, previous work in deep learning would be of great benefit.
You will collaborate with a diverse team of up to 50 international collaborators in subteams. You can join as a contributor (8-12 hours per week commitment for 10 weeks) or coach (2-4 hours per week, only for experienced DL professionals)
We’ll organise a masterclass on using the data with AirQo during the challenge to bring you up to speed.
Did you know
🗺️ 91% of the World’s Population breathes in polluted air everyday.
🫁 According to WHO, 7 million people die every year from exposure to fine particles in polluted air.
☁️ Air pollution is also damaging the health of our planet by driving climate change
Timeline
Application Deadline: 26 June 2023
Challenge Kick-off: 28 June 2023
Midterm Presentations: 26 July 2023
Final Presentations: 5 September 2023